How AI revolutionizes the world of proteins – and why this matters big time
Proteins are nature’s tools to solve a myriad of tasks. To make the human body work, evolution came up with ca. 20,000 proteins for all kinds of tasks, from fighting diseases to breaking down food. While scientists started manually reengineering proteins to make them useful for medical and industrial applications decades ago, this has been a tedious and manual task so far. Fueled by recent advances in AI, software is now taking over – reducing development cycles from years to days and giving humanity an incredibly powerful set of tools to address some of our biggest challenges. Join us for a quick briefing on the world of AI-based protein design.
Proteins are nature’s tools for almost anything
If you think about proteins, you may think about them as macro nutrients in our diet. If you are a VC or founder, you may think about food tech such as ‘alternative proteins’ for vegan alternatives to animal products. You may be vastly underestimating the relevance of these molecules.
Thousands of different proteins are integral to crucial bodily functions. They aid in the metabolism, fighting diseases, cellular signaling, and countless of other processes in all living organisms. Each protein has a very specific role it fulfills in the cells. Proteins are nature’s tools for almost everything. Think about proteins as complex functional molecules that are highly specialized for one specific purpose.
We can leverage these tools to our advantage if we understand them well enough
Nature tends to be incredibly efficient and many of humanity’s greatest inventions were inspired by natural mechanisms. If cells use proteins to solve their problems, why don’t we?
Turns out, we do. But we have not even captured 1% if the potential. Protein engineering and design has been a relevant topic for decades. However, it remains largely manual research work due to the limited understanding of how amino acid sequences relate to their structure and thus their specific functions. The cost and time associated with this research restrict teams to very targeted efforts and thereby leave >99% of theoretical solution space unexplored.
Protein design is a billion-dollar industry today – and it’s just getting started
The pharmaceutical industry invests billions in this field. The most common and typical field of application is the pharmaceutical or therapeutic field, such as drug development, including vaccines and treatments for cancer. Think about antibody-based cancer treatments (antibodies are specific proteins); think about the mRNA vaccines that taught your immune system to identify and fight the corona virus based on its spike protein.
But there is a huge industry beyond pharma as well. Novozymes, part of Europe’s most valuable company Novo Nordisk, for instance, has built billion-dollar businesses on protein design for use cases in various sectors, including chemicals, food, cosmetics, materials. Many such industrial use cases are still untapped. For most of these use cases, we talk about a group of proteins called enzymes, which act as bio-catalysts. Just like regular catalysts, they facilitate a specific chemical reaction. These bio-catalysts play a huge role in the production of all kinds of goods. With enzymatic production processes, you can manufacture compounds of unmatched purity. You can create compounds you could not create otherwise. You can manufacture commodities with unmatched efficiency, creating massive cost advantages in billion-dollar industries.
In short, designing better or fundamentally new proteins creates tremendous value. It’s a billion-dollar industry already today. But it’s characterized by tedious manual research and expensive trial-and-error. AI-based software now brings down the development cycle from years to weeks or days – a trend that will dramatically accelerate and grow the entire industry. But how can software design proteins that humans can’t?
Proteins can be designed with a programming language
Proteins are large molecules comprised of long chains of amino acids that are entangled in a delicate 3D structure. The 3D shapes of proteins determine their functions and chemical characteristics like solubility, binding affinity, or thermostability, etc.
This sequence of amino acids fully describes a protein and its resulting biochemical function. Cells produce proteins by reading out strings of RNA (an ‘executable’ copy of our DNA) bit-by-bit, where each bit encodes a specific amino acid. RNA is nature’s programming language and by specifying a specific RNA code, you can have cells (e.g., E.coli bacteria) design almost any kind of protein.
But since there is a – for all practical purposes – almost infinite number of potential amino acid sequences, designing new proteins with specific functionality is all about being smart, i.e. having a good understanding what kind of amino acid combinations will have what kind of functionality. And here is where AI and software come into play.
Biology is turning into an engineering discipline
When it comes to systematically exploring a vastly large solution space characterized by pairs of input (a protein) and output (functionality), nothing beats approaches that learn a mapping based on data. Hence, AI is poised to also dominate protein design. It’s a prime example of how biology is turning into an engineering discipline. Data and algorithms fuel a new era of engineering biochemical processes to our own benefit (often dubbed ‘tech bio’). For protein design, however, it’s a complex problem, and until recently, we lacked the basic tooling to teach AI how to solve it.
A major breakthrough in the field came in 2021 with DeepMind’s AlphaFold, when one of the most challenging problems in biochemistry, known as the protein folding problem, was solved. If you want to bet on something that will win the Nobel Prize, AlphaFold is a good candidate (Update 9. October 2024: Today, the Nobel Prize in Chemistry was indeed awarded for AlphaFold). With AlphaFold, it became possible to predict protein structures with high accuracy. The newest generation, AlphaFold3, also predicts and understands the interactions between molecules. However, this works a bit in a one-way fashion. AlphaFold shows the shape of a given amino acid sequence. It does not predict the best amino acid sequence for a desired function and thus the best protein.
This is precisely where the vast new field of AI-based protein design unfolds. It deals with the targeted solution of this problem: predicting amino acid sequences and thereby designing proteins based on desired functions. Importantly, there are many ways to go about it.
Designing proteins requires a sophisticated tech stack
Overall, there are different approaches to AI-based protein design. Some optimize and rewrite the RNA sequence directly. Interestingly, this can be based on the same technology as GPT. In a simplified way, you can think of a language model that interprets RNA sequences – or amino acid sequences – as a language (both types of sequences can be represented as a series of letters) and thereby assigns meaning to them (a so-called embedding). These models are therefore sometimes referred to as ‘Protein Language Models’. You train these models on all kinds of proteins, even unrelated to your problem, and thereby exploit massive public datasets. In a next step, you take some known proteins and map their embedding to measurements of their functionality (the test results from your wet lab), for instance with Kernel methods. Kernel methods work in both ways, so running this process in reverse give you embeddings of promising new candidates of proteins that may work even better. These methods are especially popular for optimizing existing proteins.
However, this approach is limited. Especially when you aim to design fundamentally new proteins for unsolved use cases, you may lack a good starting point for such an optimization. But there are many more sophisticated methods to design completely novel proteins beyond the aforementioned approach. Bring in AlphaFold, and you can include explicit 3D modeling of the protein structure. Bring in advanced computational chemistry, and you can narrow down what kind of 3D structures you are looking for. Bring in secret sauces of deep tech startups (that they do not want to see published), and you can come up with proteins that solve problems that no human could have ever designed manually.
AI-based protein design is currently one of the most sought-after and dynamic fields in venture capital
In this rapidly evolving field, companies like Xaira Therapeutics, Cradle, Profluent, or LabGenius, among others, are making headlines with massive funding rounds, harnessing AI to innovate and accelerate the development of new proteins. They are on a mission to make synthetic biology more accessible and cost-effective, potentially transforming how products ranging from medicines to consumer goods are developed and produced.
Xaira Therapeutics recently launched with a remarkable $1 billion in funding from Sequoia, Lightspeed and others. Leveraging advanced AI models, it aims to revolutionize the development of drugs previously deemed unattainable. Profluent also operates in the therapeutic application space and recently announced a $35 million round with Spark Capital, Insight, AIX Ventures, and Air Street Capital. In November, Index led Cradle’s Series A with a total of $24 million in new funding. LabGenius, funded by M Ventures and Atomico, recently announced their $35 million Series B.
These developments exemplify the growing VC interest in revolutionizing the pharmaceutical industry through AI-driven drug discovery, mark a significant milestone in biotechnology, and are worth taking a closer look.
Next to pharma, many startups have started taking on a pivotal role in industrial applications. Designing novel enzymes has the potential to turn billion-dollar industries upside down. Solugen, for instance, raised more than $500 million to build a specialty chemical manufacturing company that replaces petroleum-based chemicals with sustainable alternatives made from plant-based substitutes. Using enzymatic technologies and chemical solutions, Solugen creates environmentally friendly products for industries such as oil and gas, water treatment, agriculture, cleaning, and soil remediation, positioning themselves as a carbon-negative competitor to industry giants like BASF. While Solugen is a prominent example, we take a more detailed look at the tech startups dealing with enzyme design itself in this article.
To help you navigate the world of AI-based protein design startups, we have created a simple market landscape. While being far from complete, it may help you get started exploring the space. Players in the AI-based protein design companies can be clustered and characterized based along two dimensions: Use case and vertical integration.
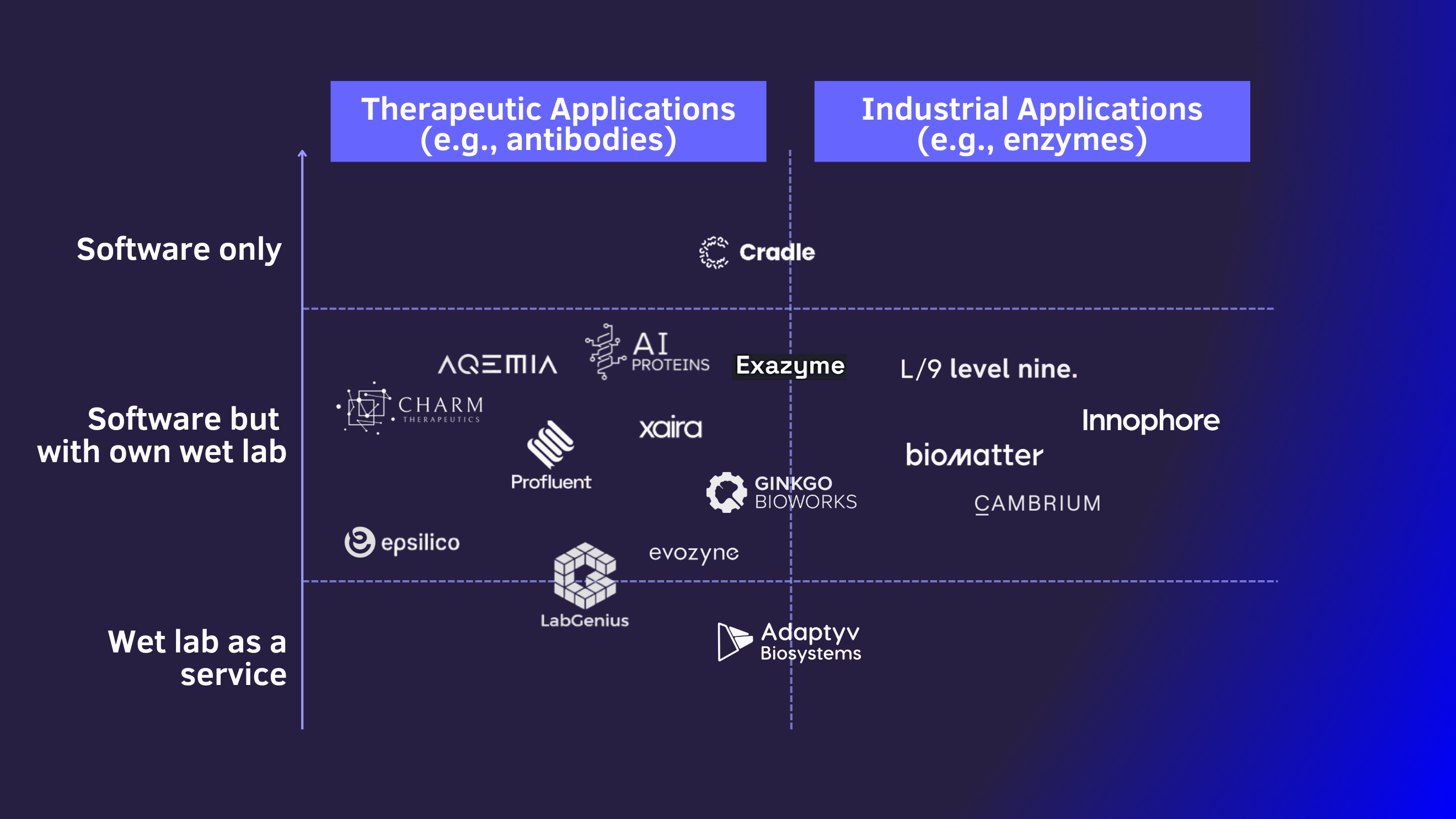
The two main distinctive areas of application for protein design are either (1) therapeutics or (2) industrial applications.
Pharmaceutical or respectively therapeutic applications mostly focus on the development of protein-based drugs such as antibodies for cancer therapies. Xaira, Cradle, and Profluent operate in this field. They mostly look to design antibodies that bind very strongly and very specifically to characteristic structures of target cells. Once they find promising candidates, the typical verification process along pre-clinical and clinical stages follows. Since such trials often cost in the billions, being smarter about choosing the right candidates to start with can create massive value. This use case is thus a part of the wider field of AI-based drug discovery (which usually deals with much simpler, smaller molecules, i.e. conventional drugs).
At least as important but less obvious are industrial applications. Examples span various sectors, including chemicals, food, cosmetics, materials, and many others. Interestingly, but not to be confused with therapeutic proteins: enzymatic production processes play an increasingly important role in mass manufacturing of drugs. While the fundamental technology to design enzymes is not too different from designing antibodies, the commercial nature of startups in this field differs a lot. While we see all different kinds of business models (from SaaS to IP licensing), these startups typically do not need to go through lengthy certification processes with their proteins as their target industries are much less regulated.
The depth of vertical integration is pivotal for protein design startups
The vertical integration strongly determines the value created and captured by the startup. As always, there are different schools of thought on what’s best. But either way, it is for definitely worth discussing it.
This article largely makes the case that AI-based software is starting to dominate protein design. But the interface to the physical world is crucial. AI learns from feedback cycles, so you need to get measurements from a physical wet lab, in which proteins are produced and characterized, into your software for it to improve. Doing this in-house brings in complexity and goes along with substantial costs, but it also generates proprietary insights and data sets.
Some companies focus solely on this part, running wet labs for protein characteristics as a service. Other companies solely focus on building software that designs proteins and outsource all physical things to partners or customers. VCs may tend to shy away from the physical world, naturally preferring pure-play software companies. But here, they should think twice. We see that most founders realized that in order to iterate fast, in order to bring in all needed competencies, and in order to capture the value their software creates, they need to run their own wet lab. However, only few companies combine all the required skills in their founding team and only few companies manage to raise the cash in order to afford this approach in their early stages.
We found that those with their own proprietary wet labs, which enable rapid testing and iteration of candidates, appear most promising. These labs allow for efficient operations and the maintenance of closer relationships with their clients, including IP ownership and the specific use cases for the designed proteins. Hence, relevant value creation and, more importantly, value capture can only be achieved by mastering the full stack.
Let us hear your thoughts!
The field of AI-based protein design is incredibly fast-moving, promising, and complex alike. This article is meant as a discussion starter, outlining UVC Partners’ current thinking. We appreciate that the article quite drastically simplifies many things and is far from complete – neither in terms of technology trends nor in terms of companies mentioned. Let us know what you think, what we missed, and what you see differently!
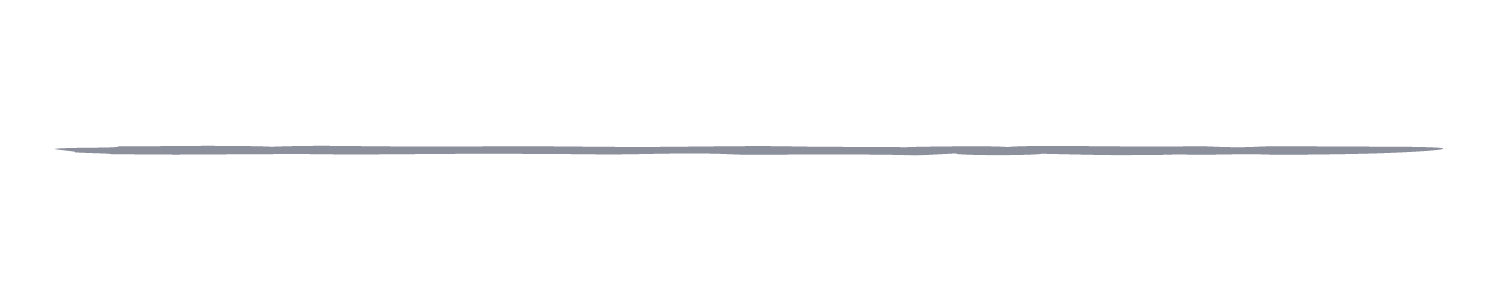
About the authors

Jackie Kroyer is an investor with UVC Partners’ Berlin office, specializing in software and AI startups. She holds a Master's degree in Management & Technology from TU Munich with a focus on informatics and finance and has a strong passion for diving deep into Chem Tech-related domains, such as Bio Tech or Energy Tech.
Mail: jackie.kroyer@uvcpartners.com
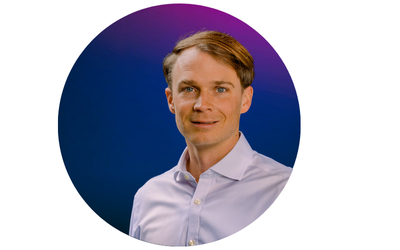
Dr. Oliver Schoppe is a principal with UVC Partners’ Munich Office. He spent his academic education at the interface of AI, biology, and medicine. At UVC Partners, he focuses on AI and software startups.
Mail: schoppe@uvcpartners.com
About UVC Partners
UVC Partners is a leading Munich- and Berlin-based early-stage venture capital firm that invests in European B2B tech startups from pre-seed to series A. With about €400 million in assets under management, UVC Partners typically invests between €500,000 and €10 million initially and up to €30 million per company. The portfolio includes category leaders in deep tech, climate tech, hard- and software, and mobility with various technologies and business models. As an independent partner of UnternehmerTUM, Europe's most extensive innovation and startup center, UVC Partners has access to proprietary deal flow, an industrial network of more than 1,000 corporates, and access to talent from the leading European technical university. The investments include Flix, Vimcar, planqc, Tanso, Isar Aerospace, TWAICE, DeepDrive, STABL, and many more. They all benefit from the team's extensive investment and exit experience, their ability to build sustainable category leaders with a competitive advantage, and their passion for growing the game changers of tomorrow.